A Review article on Consciousness was recently published in The Journal of Neuroscience (8 Nov. 2017).
“Consciousness Regained: Disentangling Mechanisms, Brain Systems, and Behavioral Responses”
Johan F. Storm, Mélanie Boly, Adenauer G. Casali, Marcello Massimini, Umberto Olcese, Cyriel M.A. Pennartz and Melanie Wilke
The Journal of Neuroscience, 8 November 2017, 37(45):10882-10893;doi:10.1523/JNEUROSCI.1838-17.2017
(http://www.jneurosci.org/content/37/45/10882?etoc=)
Abstract:
How consciousness (experience) arises from and relates to material brain processes (the “mind-body problem”) has been pondered by thinkers for centuries, and is regarded as among the deepest unsolved problems in science, with wide-ranging theoretical, clinical, and ethical implications. Until the last few decades, this was largely seen as a philosophical topic, but not widely accepted in mainstream neuroscience. Since the 1980s, however, novel methods and theoretical advances have yielded remarkable results, opening up the field for scientific and clinical progress. Since a seminal paper by Crick and Koch (1998) claimed that a science of consciousness should first search for its neural correlates (NCC), a variety of correlates have been suggested, including both content-specific NCCs, determining particular phenomenal components within an experience, and the full NCC, the neural substrates supporting entire conscious experiences. In this review, we present recent progress on theoretical, experimental, and clinical issues. Specifically, we (1) review methodological advances that are important for dissociating conscious experience from related enabling and executive functions, (2) suggest how critically reconsidering the role of the frontal cortex may further delineate NCCs, (3) advocate the need for general, objective, brain-based measures of the capacity for consciousness that are independent of sensory processing and executive functions, and (4) show how animal studies can reveal population and network phenomena of relevance for understanding mechanisms of consciousness.
Figures:
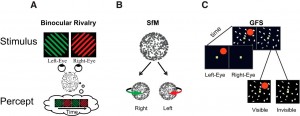
Examples of bistable stimuli. A, In binocular rivalry, two stimuli are shown to different eyes and perception wavers between left and right eye stimuli (Blake and Logothetis, 2002). B, Ambiguous structure-from-motion (SfM) stimulus. Dots moving back and forth on a flat screen, without perspective cues to differentiate between front and rear surfaces, induce the perception of a 3D rotating object that periodically switches direction. (Sterzer et al., 2009) C, Generalized flash suppression. A target stimulus (red dot) is shown parafoveally followed by the onset of a moving surround, causing the red target to disappear in ∼50% of trials (Wilke et al., 2003).
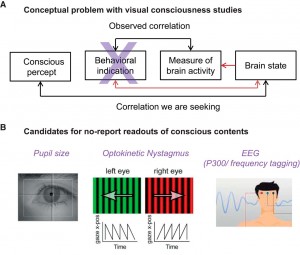
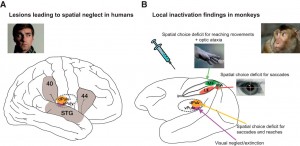
A, Major cortical and subcortical brain regions where lesions lead to spatial neglect in humans (left), and corresponding recent experimental results in monkeys (right) (lateral view). In humans, lesions in frontal (Brodmann area 44), inferior parietal cortex (Brodmann area 40), superior temporal gyrus (STG), basal ganglia, and pulvinar have been reported to lead to spatially biased behavior that might appear as a visual consciousness deficit (Karnath, 2001). B, Recent pharmacological inactivation studies in monkeys have shown primarily effector-specific spatial deficits after lesions in parietal subregions such as the lateral intraparietal area (LIP, red shading) and the parietal reach region (PRR, which includes the medial intraparietal area MIP and area V6A, green shading). Dorsal pulvinar (dPULV, orange shading) inactivation leads to spatial orienting bias for both eye and hand movements which can be compensated by visual reward cues, suggesting that visual perception might be preserved. Ventral pulvinar (vPULV, purple shading) inactivation leads to change detection deficits resembling visual neglect. Summarized from local inactivation studies in monkeys (Wardak et al., 2002; Wilke et al., 2010; Hwang et al., 2012; Wilke et al., 2012; Wilke et al., 2013; Hwang et al., 2014; Christopoulos et al., 2015; Katz et al., 2016; Zhou et al., 2016).
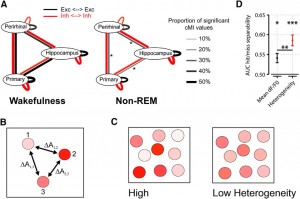
A, Summary of main findings on spike-based functional connectivity in rats (Olcese et al., 2016). Coupling was measured as pairwise cMI between single neurons. During wakefulness, cMI between neurons located in the same or different areas is largely balanced (left) for both excitatory and inhibitory neurons (black and red lines, respectively). In NREM sleep, interareal (but not intra-area) coupling between excitatory neurons is significantly reduced. This did not apply to intra-area cMI and interareal cMI (between interneurons). Line thickness indicates the proportion of neuronal pairs for which cMI was significantly >0. Asterisks indicate which connections showed a significant change between wakefulness and NREM sleep (the only significant differences found pertained to interareal coupling between excitatory neurons). Thus, during NREM sleep, neural computations may continue in local “islands of activity,” whereas global integration capabilities are reduced. B, Calculation of heterogeneity across a neuronal population (compare Montijn et al., 2015). A measure of a neuronal activity change (A, e.g., the relative fluorescence response of a neuron in 2-photon imaging, dF/F0) is computed across all neurons. Next, the responses are z-scored per neuron across all trials and all trial types (e.g., in a given session, visual stimuli are presented at 6 different contrasts; each contrast is presented 20 times; 120 trials in total). Per trial, the absolute difference in z-scored activity is then calculated across all pairs of neurons (e.g., ΔA1,2 is the difference between the responses of neurons 1 and 2). The population heterogeneity in a given trial is the mean of activity differences across all pairs. C, Examples of high (left) versus low heterogeneity (right) in a neuronal population, where response strength is indicated by color saturation. D, In a visual stimulus detection task performed by mice that were subjected to 2-photon imaging of V1 neuronal populations, heterogeneity was better capable of separating hit (detection) and miss (nondetection) trials than the mean fluorescence response (area under the curve resulting from receiver-operating characteristic analysis). Both measures predicted response behavior above chance: mean response, *p < 0.05; heterogeneity, ***p < 0.001; area under the curve = 0.5, chance level. Behavior was predicted better by heterogeneity than mean response (**p < 0.01). Values are mean ± SEM across animals. Data from Montijn et al. (2015).